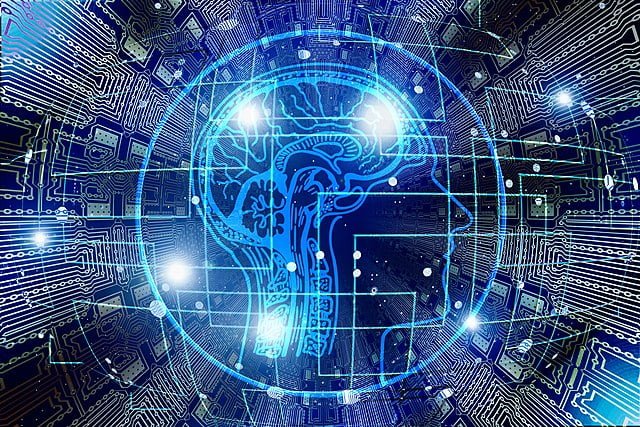
The discourse surrounding Large Language Models (LLM) and their potential to be intelligent agents has been a focal point in discussions about these powerful Artificial Intelligence (AI) systems. However, a recent study suggests that AI lacks the crucial human capacity for innovation.
While both children and adults can solve problems by finding novel uses for everyday objects, artificial intelligence systems cannot often see tools in a new light, as concluded by researchers at the University of California, Berkeley.
A new perspective is emerging, focusing on the cultural importance of AI and its ability to drive innovation and cultural transmission.
AI as Cultural Technologies
Rather than framing LLM solely as intelligent entities, this new perspective considers them “cultural technologies.” By efficiently analyzing and processing vast amounts of text and data, these models act as powerful engines for cultural transmission, disseminating knowledge, ideas, and artistic expressions across generations. Their ability to learn and adapt to new information allows them to continuously refine and update our cultural landscape.
However, the potential of LLM goes beyond mere cultural transmission. Some experts believe they can unlock new insights into the fundamental processes of “imitation and innovation.” By comparing LLM responses with those of human children when facing novel situations and tasks, researchers can gain valuable insights into the cognitive mechanisms driving learning and creativity.
AI vs. Children
As part of their study, researchers tested the ability of artificial intelligence systems to imitate and innovate compared to that of children and adults.
In one experiment, 42 children (aged 3 to 7) and 30 adults were presented with textual descriptions of everyday objects. In the first part, 88% of children and 84% of adults could correctly identify which objects would “pair best” with another. For instance, they paired a compass with a ruler instead of a teapot.
In the next stage, 85% of children and 95% of adults could also innovate the expected use of everyday objects to solve problems. In one task, participants were asked how they could draw a circle without using a typical tool like a compass. Faced with choices like a similar tool such as a ruler, a different tool like a round-bottomed teapot, and an irrelevant tool like a stove, most participants chose the teapot, a conceptually different tool that could still achieve the same goal by functioning as a compass, allowing them to trace the shape of a circle.
When the same textual descriptions were provided to five large language models, the models performed similarly to humans in the imitation task, with scores ranging from 59% for the worst-performing model to 83% for the best-performing model. However, AI responses to the innovation task were much less accurate. Effective tools were selected 8% of the time by the worst-performing model and 75% by the best-performing model.
“Children can imagine completely novel uses for objects they have never seen or heard of before, like using the bottom of a teapot to draw a circle,” said Yiu. “Large models find it much more challenging to generate these kinds of responses.”
Imitation or Innovation?
This line of research has immense potential to understand the intricate relationship between imitation, a crucial skill for learning existing knowledge, and innovation, the ability to generate new ideas and solutions. LLMs can serve as powerful tools to explore the limits of these cognitive processes and to identify the specific representations, competencies, and knowledge required for each.
Furthermore, examining the results of LLMs trained with large-scale linguistic data allows us to explore the limits of statistical analysis to drive innovation. While LLMs excel at acquiring and reproducing existing knowledge, our current understanding suggests they may lack the crucial ingredients for the type of independent and creative thinking displayed by human children.
This observation suggests that achieving true innovation may require more than simply inputting vast amounts of data into AI systems. It may require alternative learning paradigms that incorporate elements such as bodily experiences, social interaction, and the ability to reason about causality.
Conclusion
“AI can help transmit information that is already known but is not innovative,” said Yiu. “These models can summarize conventional wisdom, but they cannot expand, create, change, abandon, evaluate, and improve conventional wisdom the way a young human can.” However, AI development is still in its early stages, and there is much to learn about how to expand AI’s learning capacity, Yiu affirmed. Drawing inspiration from children’s curious, active, and intrinsically motivated learning approach could help researchers design new AI systems better equipped to explore the real world.
In conclusion, exploring AI through a cultural and cognitive lens offers a new perspective on its potential beyond the bounds of intelligence. By recognizing its role in cultural transmission and its ability to contribute to our understanding of imitation and innovation, we can pave the way for the development of truly innovative and transformative AI systems. The future of AI lies not only in replicating intelligence but in harnessing its potential to enhance our culture, understanding, and creative capabilities.
Contact
Eunice Yiu
Department of Psychology, University of California, Berkeley
Email: ey242@berkeley.edu
Reference (open access)
Yiu, E., Kosoy, E., & Gopnik, A. (2023). Transmission Versus Truth, Imitation Versus Innovation: What Children Can Do That Large Language and Language-and-Vision Models Cannot (Yet). Perspectives on Psychological Science, 0(0). https://doi.org/10.1177/17456916231201401