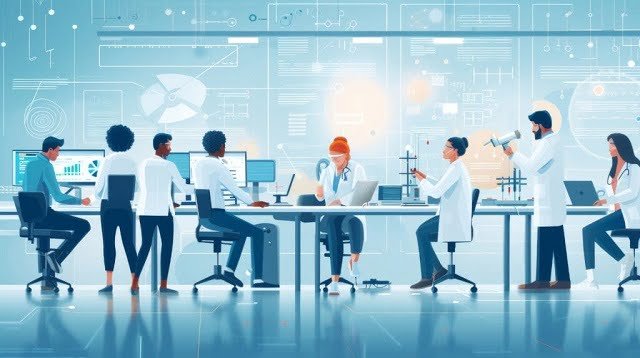
Machine Learning (ML), a type of artificial intelligence, is rapidly transforming scientific research and offering powerful tools for discovering hidden patterns and accelerating discovery; such as finding early disease markers and aiding policymakers in avoiding decisions leading to war.
However, the increased adoption of ML has not been without challenges. Studies have revealed issues with the validity, reproducibility, and generalization of some ML-based research. This can lead to wasted resources, unreliable scientific conclusions, and ultimately, a loss of trust in machine learning as a scientific method.
The good news? Researchers are actively working on solutions. Recognizing that similar obstacles often affect ML applications across various disciplines, a team of experts from Princeton University (USA), Cornell University (USA), Duke University (USA), Norwegian University of Science and Technology (Norway), among other academic organizations, has developed a comprehensive framework to guide solid and reliable ML-based science. This framework, called REFORMS (Recommendations for Machine Learning-Based Science), provides a valuable checklist to ensure the quality and reliability of your research.
‘When we move from traditional statistical methods to machine learning methods, there are many more ways to shoot ourselves in the foot,’ said Arvind Narayanan, director of the Center for Information Technology Policy at Princeton University and computer science professor. ‘If we don’t have an intervention to improve our scientific and reporting standards regarding machine learning-based science, we risk not only one discipline but many different scientific disciplines rediscovering these crises one after another.’
The Problem: Errors Plague Machine Learning-Based Science
The rapid adoption of machine learning methods has exposed vulnerabilities in how these studies are conducted and reported. Common issues include:
- Difficulty accurately evaluating model performance.
- Complex and non-standardized ML code, making replication difficult.
- Confusing explanatory and predictive models, leading to misinterpreted results.
- Excessive optimism about ML capabilities, which could bias research.
These problems create a ‘feedback loop’ where unreliable findings are cited more frequently, further perpetuating misconceptions.
Because machine learning has been adopted in virtually every scientific discipline without universal standards safeguarding the integrity of those methods, Narayanan said the current crisis, which he calls a reproducibility crisis, could become much more severe than the replication crisis that emerged in social psychology over a decade ago.
REFORMS: A Roadmap for Responsible Machine Learning in Science
REFORMS is a consensus-driven effort meticulously crafted by a team of 19 researchers with expertise in computer science, data science, mathematics, social sciences, and biomedical sciences. This diverse group pooled their knowledge to create a practical tool for researchers, reviewers, and journal editors alike.
The REFORMS checklist consists of 32 key questions paired with corresponding guidelines. By addressing these questions throughout the research process, you can significantly enhance the transparency, reproducibility, and overall robustness of your ML-based studies. Here’s a glimpse into some of the areas REFORMS addresses:
- Data Quality: REFORMS emphasizes the importance of examining your data for biases, inconsistencies, and missing values.
- Model Selection and Evaluation: Choosing the right ML model is crucial. REFORMS helps you navigate this selection process and ensure proper model evaluation through solid metrics.
- Generalizability and Overfitting: Generalization refers to your model’s ability to perform well with unseen data. REFORMS provides methods to mitigate overfitting, a common issue when a model memorizes the training data and fails to generalize to new situations.
- Transparency and Reporting: Clear and concise reporting is essential for scientific progress. REFORMS guides how to document your research methods, model choices, and results in a way that enables independent evaluation and replication.
The checklist focuses on ensuring the integrity of research using machine learning. Science relies on the ability to reproduce results independently and validate claims. Otherwise, new work cannot be reliably built upon old, and the entire enterprise will collapse. While other researchers have developed checklists that apply to problems in specific disciplines, especially in medicine, the new guidelines start with underlying methods and apply them to any quantitative discipline.
REFORMS: Empowering Researchers, Reviewers, and Editors
The REFORMS checklist is a valuable resource for all stakeholders in the field of ML-based science:
- Researchers: Use REFORMS to design and implement robust, transparent, and reproducible studies. This will strengthen the foundation of your research and enhance its potential impact. The checklist asks researchers to provide detailed descriptions of each machine learning model, including code, data used for training and testing the model, hardware specifications used to produce the results, experimental design, project goals, and any limitations of the study findings.
- Reviewers: REFORMS provides you with a comprehensive framework for critically assessing the quality and rigor of ML-based research papers.
- Journal Editors: By adopting REFORMS as a benchmark, journals can promote higher standards of transparency and reproducibility in published research.
Benefits of Using REFORMS
By adopting REFORMS, the scientific community can gain significant benefits:
- Enhanced Credibility: REFORMS instills confidence in ML research by promoting robust methodologies and transparent reporting.
- Reduction of Time and Resource Loss: By ensuring studies are designed to be replicable, REFORMS helps researchers avoid wasted time and resources.
- Accelerated Progress: REFORMS facilitates the development of existing research, leading to faster scientific advances.
- Improved Collaboration: By establishing a common framework, REFORMS promotes collaboration across disciplines, fostering innovation.
The Future of Reliable Machine Learning in Science
By adopting robust methodologies and fostering a culture of transparency, researchers can unlock the true potential of machine learning in scientific discovery. REFORMS serves as a beacon guiding the way toward reliable and impactful ML-based science. As this field continues to evolve, REFORMS will undoubtedly play a vital role in ensuring its responsible and reliable application across the scientific landscape.
While the increased rigor of these new standards might slow the publication of any given study, the authors believe widespread adoption of these standards would increase the overall rate of discovery and innovation, potentially by a large margin.
‘What we ultimately care about is the pace of scientific progress,’ said sociologist Emily Cantrell, one of the lead authors, who is pursuing her Ph.D. at Princeton. ‘By making sure that articles that are published are of high quality and that they are a solid foundation on which to build future articles, that could potentially accelerate the pace of scientific progress. Our emphasis should be on focusing on scientific progress itself and not just on publishing articles.’
Referencia (acceso abierto)
Kapoor, S., Cantrell, E. M., Peng, K., Pham, T. H., Bail, C. A., Gundersen, O. E., Hofman, J. M., Hullman, J., Lones, M. A., Malik, M. M., Nanayakkara, P., Poldrack, R. A., Raji, I. D., Roberts, M., Salganik, M. J., Serra-Garcia, M., Stewart, B. M., Vandewiele, G., & Narayanan, A. (2024). REFORMS: Consensus-based Recommendations for Machine-learning-based Science. Science Advances. https://doi.org/adk3452
Note: Prepared with information from the press release and the scientific article.