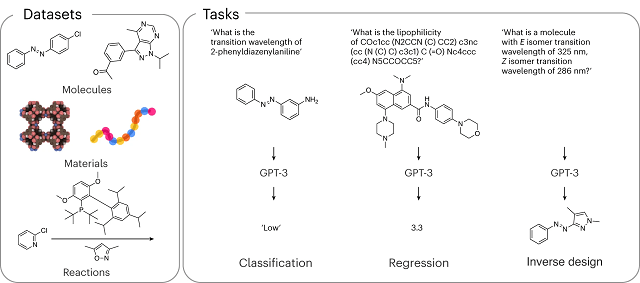
Imagine exploring the vast realms of chemistry and materials science not only through formulas and equations but also through the power of natural language.
Artificial intelligence is becoming a fundamental tool in chemical research, offering novel methods to tackle complex challenges that traditional approaches struggle with. One subtype of artificial intelligence that has been increasingly utilized in chemistry is machine learning, which employs algorithms and statistical models to make data-driven decisions and perform tasks for which it hasn’t been explicitly programmed.
However, to make reliable predictions, machine learning also demands large amounts of data, which are not always available in chemical research. Small chemical datasets simply do not provide enough information for these algorithms to train on, limiting their effectiveness.
In a new study, scientists from Berend Smit’s team at Ecole Polytechnique Federale de Lausanne (EPFL) have found a solution in large language models like GPT-3. These models are pre-trained on massive amounts of text and are known for their broad capabilities to understand and generate human-like texts. GPT-3 forms the basis of the most popular AI, ChatGPT.
GPT-3
The study, published in Nature Machine Intelligence, unveils a novel approach that significantly simplifies chemical analysis using artificial intelligence. Contrary to initial skepticism, the method doesn’t directly pose chemical questions to GPT-3. “GPT-3 hasn’t seen most of the chemical literature, so if we ask ChatGPT a chemical question, the answers are usually limited to what can be found on Wikipedia,” says Kevin Jablonka, the study’s lead researcher. “Instead, we fine-tune GPT-3 with a small dataset converted into questions and answers, creating a new model capable of providing accurate chemical information.”
This process involves feeding GPT-3 a selected list of questions and answers. “For example, for high-entropy alloys, it’s important to know if an alloy exists in a single phase or has multiple phases,” says Smit. “The selected list of questions and answers is of the form: Q=’Is it a single phase?’ A=’Yes/No’.”
And he continues: “In the literature, we’ve found many alloys whose answer is known, and we use this data to fine-tune GPT-3. What we get is a refined AI model that’s trained to answer this question solely with a yes or a no.”
Study Results
In tests, the model, trained with relatively few questions and answers, correctly answered over 95% of highly diverse chemical problems, often surpassing the accuracy of state-of-the-art machine learning models. “The thing is, this is as easy as doing a literature search, which works for many chemical problems,” says Smit.
Here’s why this discovery is so exciting:
- Small data, big ideas: Traditionally, machine learning in chemistry has been hindered by the limited size of chemical datasets. This new approach overcomes this barrier by leveraging GPT-3’s vast knowledge extracted from the internet.
- Ask, and the model will answer: No complex coding or specialized knowledge is required. Researchers can simply pose questions in natural language to GPT-3, and it provides accurate answers about material properties, synthesis, and design. Imagine asking, “What’s the most efficient way to create a material with specific optical properties?” and receiving a data-driven response.
- Surpassing traditional techniques: It’s not just about convenience. The study revealed that GPT-3, when fine-tuned, can match or even outperform dedicated machine learning models, especially for smaller datasets. This opens up possibilities for rapid exploration and discovery in areas with limited data.
- Simplified reverse design: Want to design a material with specific properties? Simply “reverse” your question. Instead of asking about the properties of a material, ask about the material exhibiting the desired properties. This opens the doors to a completely new pathway of designing specific materials.
- Democratizing scientific discovery: The ease of use and impressive performance, especially with limited data, have the potential to empower researchers at all levels. Anyone with a curious mind and a question can tap into GPT-3’s vast knowledge base, making scientific exploration more accessible and collaborative.
Implications for scientific research
One of the most striking aspects of this study is its simplicity and speed. Traditional machine learning models require months to develop and demand extensive expertise. In contrast, the method developed by Jablonka takes five minutes and requires no prior knowledge.
The implications of the study are profound. It introduces a method as simple as conducting a literature search, applicable to various chemical problems. The ability to ask questions like “Is the performance of a [chemical] made with this [recipe] high?” and receive accurate answers could revolutionize how chemical research is planned and conducted.
Conclusion
In the paper, the authors state: “In addition to a literature search, querying a foundational model [e.g., GPT-3,4] could become a routine way to initiate a project by harnessing collective knowledge encoded in these foundational models.” Or, as Smit succinctly puts it, “This is going to change how we do chemistry.”
While challenges remain, such as ensuring scientific rigor and interpretability of results, this research marks a significant step forward. As scientists continue to unlock the potential of large language models in chemistry and beyond, one thing is clear: The future of scientific exploration is driven by both data and the power of words.
Lastly, while GPT-3 is impressive, it’s still in development. Always critically evaluate its results and consult with experts for complex tasks.
The study was funded by the Swiss National Science Foundation, Grantham Foundation for the Protection of the Environment, RMI Third Derivative, and Carl Zeiss Foundation.
Contact
Berend Smit
Laboratory of Molecular Simulation (LSMO), Institut des Sciences et Ingénierie Chimiques
École Polytechnique Fédérale de Lausanne (EPFL), Sion, Switzerland
Email: berend.smit@epfl.ch
Reference (open access)
Jablonka, K.M., Schwaller, P., Ortega-Guerrero, A. et al. Leveraging large language models for predictive chemistry. Nat Mach Intell (2024). https://doi.org/10.1038/s42256-023-00788-1
Note: Compiled with information from Ecole Polytechnique Federale de Lausanne (EPFL) press release and the scientific article.