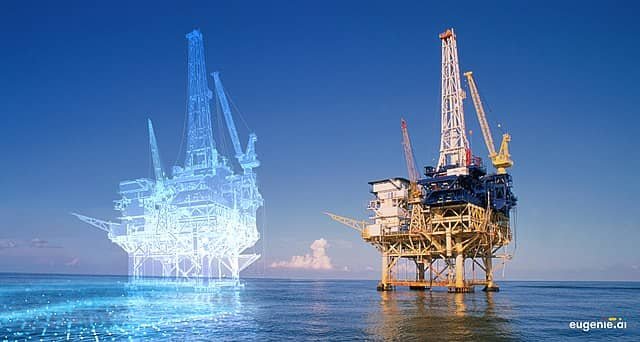
Technology is rapidly advancing and transforming how we design, manage, and optimize complex systems. In this context, the Digital Twin has emerged as a revolutionary tool, capable of digitally replicating physical objects and processes to predict behaviors, optimize outcomes, and solve problems in real time. But what exactly is a Digital Twin, how does it work, and what are its applications? If your company is undergoing a digital transformation process, you should know that digital twins should play a significant role in designing and optimizing your products or services.
The increasing use of artificial intelligence, machine learning, and the Internet of Things, along with the ongoing automation of various industries, is driving entrepreneurs to implement digital twins in their processes to reduce time-to-market.
Currently, the role of digital twins is expanding in manufacturing to include product design, process optimization, quality management, supply chain management, predictive maintenance, interdisciplinary collaboration, and customer experience (Crawford, 2021).
Digital twins (virtual replicas of machines or systems) are revolutionizing industries (Tao and Qi, 2019). According to a study by Grand View Research Inc., the global market for digital twin applications is expected to reach a value of $86 billion by 2028.
This article aims to provide you with a definition of a digital twin, its types, applications, and some examples to help you understand the potential of this tool for your business.
What is a Digital Twin?
The concept of a “Digital Twin” was introduced in 2003 by Michael Grieves (Grieves, 2014) and published by NASA in 2012 (Glaessgen and Stargel, 2012). Digital twin modeling is at the core of accurately representing a physical entity, enabling it to deliver functional services and meet application requirements (Tao et al., 2022).
Digital twins integrate all data (tests, operational data, etc.), models (designs, engineering models, analyses, etc.), and other information (requirements, orders, inspections, etc.) of a physical object generated throughout its lifecycle to seize business opportunities (Boschert y Rosen, 2016).
IBM defines a digital twin as “a virtual representation of an object or system that spans its lifecycle, is updated from real-time data, and uses simulation, machine learning, and reasoning to aid decision-making.” In summary, a digital twin is an accurate digital representation of a physical object, system, or process. Through real-time data, mathematical models, and simulations, it allows for the analysis, prediction, and optimization of the behavior of its physical counterpart. Essentially, it acts as a “digital mirror” that enhances understanding and decision-making.
Digital twins integrate all the information and knowledge generated during a product’s lifecycle, from its conception and design to its end of life (Hartmann y Van der Auweraer, 2020). This way, a product’s digital twin can contribute to the circular economy.
Key Features of Digital Twin Technology:
- Real-time data integration: Live updates from sensors on the physical asset.
- Advanced analytics: AI and machine learning for predictive insights.
- Lifecycle support: Covers design, operation, and end-of-life phases.
How Does Digital Twin Technology Work?
Digital twin technology operates by collecting sensor data and integrating it with advanced computational models. The process can be divided into three main stages:
- Creating the digital twin: Using software like CAD, a detailed 3D model is constructed. Powered by real-time sensor data, computational models generated by digital twins replicate every aspect of a product, process, or service (Tao and Qi, 2019).
- Real-time data collection: IoT sensors monitor the state of the physical object. These sensors generate data on various aspects of the object’s performance, such as energy production, temperature, weather conditions, and more. This data is then linked to a processing system and applied to the digital copy.
- Analysis and simulation: Algorithms process the data to identify patterns, predict failures, and optimize operations. Once loaded with data, the virtual model can be used for simulations, performance studies, and potential improvements to gain valuable insights that can then be applied to the physical object.
Digital Twins vs. Simulations
Although the terms Digital Twin and Simulation are often confused, there are clear differences. While both use digital models to replicate system processes, a digital twin is a virtual environment connected to its physical counterpart and operates in real time with constantly updated data.
- Simulation: A generic computational model that represents a process under specific conditions.
- Digital Twin: A digital replica connected to its physical counterpart, operating in real-time and dynamically updated.
According to IBM, the difference lies in scale: a simulation typically focuses on a specific process, whereas a digital twin can develop multiple simulations to study different processes. Digital Twins surpass traditional simulations by offering dynamic and predictive precision, making them more useful in complex applications like critical infrastructure management.
Digital Twin vs. Virtual Twin: Which is Better?
Another common debate is Digital Twin vs. Virtual Twin. While a Digital Twin reflects real-time data, a Virtual Twin is a conceptual representation that is not necessarily tied to current data.
Both have their place depending on the use case. A Virtual Twin is ideal for the early design stage, whereas a Digital Twin is essential for continuous operation and maintenance.
Types of Digital Twins
There are various types of Digital Twins, depending on the level of product scaling. IBM notes that the primary difference between Digital Twins lies in their area of application.
Component Twins/Part Twins
Component Twins represent the smallest unit of a Digital Twin, focusing on individual functional components. Part Twins are similar but refer to components of slightly lesser importance.
Asset Twins
When two or more components work together, they form what is known as an asset. Asset Twins allow you to study the interaction between these components, generating performance data that can be processed into actionable insights.
System or Unit Twins
The next level involves System or Unit Twins, which provide an overview of how various assets come together to form a fully functional system. These twins offer visibility into asset interactions and suggest performance improvements.
Process Twins
At the macro level, Process Twins reveal how systems work together to create an entire production facility. This type of Digital Twin can help determine precise scheduling, ultimately impacting overall efficiency.
Advantages of Using Digital Twins
It is widely acknowledged that Digital Twins lead to cost savings throughout the entire lifecycle while enabling new services such as diagnostics, preventive maintenance, or operational optimization (Van der Auweraer et al., 2018).
Jiang et al (2021) highlight that, with the support of Digital Twin techniques, modern industries can perform a wide range of online tasks, covering R&D, manufacturing and assembly, as well as marketing and sales.
IBM also outlines the following benefits of Digital Twins:
Enhanced Research and Development (R&D)
Digital Twins enable more effective research and product design by generating vast amounts of data on potential performance outcomes. This information can yield insights that help companies refine products before beginning production.
Tao and Qi (2019) suggest the following steps for more consistent Digital Twin R&D:
- Standardize data and models.
- Share data and models.
- Innovate in services.
- Establish forums.
Increased Efficiency
Even after a new product enters production, Digital Twins can help monitor and reflect production systems to achieve and maintain maximum efficiency throughout the manufacturing process.
According to Soori et al., (2023), by simulating manufacturing processes and identifying potential bottlenecks and inefficiencies, manufacturers can optimize operations, increase productivity, and reduce waste.
End of Product Lifecycle
Digital Twins can assist manufacturers in determining what to do with products that reach the end of their lifecycle and require final processing, such as recycling or other measures.
By using Digital Twins, manufacturers can identify which product materials can be collected for reuse.
Examples of Digital Twin Applications
Digital Twin tools are being employed across various sectors, including industry, agriculture, livestock, and healthcare. Below is an overview of their applications and some examples:
Manufacturing Operations
Digital Twins are key tools for addressing today’s industrial challenges (Hartmann and Van der Auweraer, 2021). Virtual models promote smart manufacturing by simulating decisions and optimizing processes (Tao y Qi, 2019). Production teams can analyze multiple data sources to reduce defective items, improve production efficiency, and minimize industrial downtime (Soori et al., 2023).
IBM emphasizes that since Digital Twins are designed to reflect the entire lifecycle of a product, it is no surprise that they have become ubiquitous across all stages of manufacturing—guiding products from design to final production and every step in between.
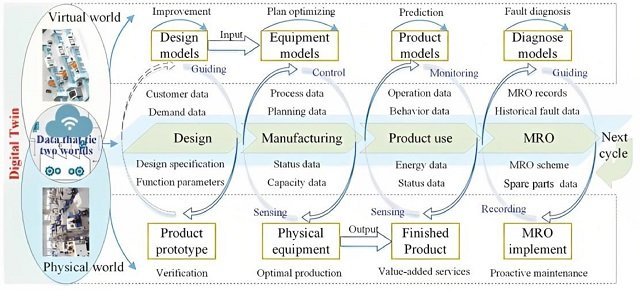
Healthcare Services
Just as products can be profiled using digital twins, patients receiving healthcare services can also be profiled. Digital twins can optimize clinical operations by analyzing workflows and resource allocation, leading to streamlined processes and improved patient care (Vallée, 2023).
A digital twin can also be used for personalized medicine services. Björnsson et al. (2019) explain that personalized medicine requires the integration and processing of vast amounts of data and propose a solution based on constructing digital twins.
Chow (2021) highlights a team of doctors and technologists in Poland who developed a digital twin of a fetal heart called Fetal Heart VR. This tool allows physicians to guide a probe through a womb-like dome to study fetal hearts with both normal and abnormal rhythms.
Similarly, Voigt et al. (2021) discuss the use of digital twins for multiple sclerosis as a revolutionary tool to improve diagnosis, monitoring, and therapy. This approach saves costs and helps prevent disease progression.
Power Generation Equipment
Large engines, including jet engines, locomotive engines, and power generation turbines, benefit greatly from digital twins, particularly for establishing regular maintenance schedules.
Structures and Systems
Large physical structures, such as high-rise buildings or offshore drilling platforms, can be enhanced through digital twins, especially during their design phase. Digital twins are also valuable for designing systems operating within these structures, such as HVAC systems.
Automotive Industry
Vehicles are complex systems with numerous interconnected components, making digital twins invaluable for car design. They improve vehicle performance and increase production efficiency.
Chow (2021) mentions BMW’s Regensburg factory, which features a physical plant and a 3D virtual replica accessible via VR headsets.
Urban Planning
Civil engineers, architects, and urban planners benefit significantly from digital twins, which can display 3D and 4D spatial data in real time and incorporate augmented reality systems into built environments.
On a larger scale, digital twins are being created for entire cities and even countries (Chow, 2021). For example, Singapore is developing a digital replica of the entire city to monitor and improve services (Tao and Qi, 2019).
Urban planners use digital twins to:
- Model traffic flows and optimize public transport.
- Simulate infrastructure performance under extreme weather conditions.
- Integrate sustainable practices into city development.
Infrastructure Management and Maintenance
Digital twins are used in managing and maintaining public and private infrastructure. Esteban (2021) notes that integrating BIM infrastructure models with cartographic and geographic environments supported by GIS systems enables dynamic systems connected in real time to real-world scenarios.
Smart Farming and Agriculture
Verdouw et al (2021) highlight the promise of digital twins in achieving new levels of productivity and sustainability in smart farming. Producers can remotely manage farm operations based on real-time digital information, enabling immediate action for deviations and simulations of intervention effects.
Verdouw et al. validated their approach through five smart farming cases in the European IoF2020 project, focusing on arable farming, dairy farming, greenhouse horticulture, organic farming, and livestock farming.
Pylianidis et al (2021) emphasize the use of agricultural digital twins at various spatial and temporal scales and propose a roadmap for their adoption in agriculture.
Circular Economy
Preut et al. (2021) discuss digital twins’ contributions to product circularity and managing circular supply chains. However, they note the need for further research, particularly for product-specific implementations.
Smart Aquaculture
Lima et al (2022) propose using a digital twin for managing a land-based rainbow trout farm. The approach is based on precision aquaculture management phases, including sensors, Big Data, the Internet of Things, and predictive mathematical models.
Digital Twin as a Service: Cloud Innovation
The democratization of technology is driving the Digital Twin as a Service model. Platforms like AWS IoT TwinMaker provide tools for businesses of all sizes to implement digital twins in the cloud, avoiding costly upfront investments.
Tao et al., (2024) proposed and designed a reference architecture for a digital twin software platform called “makeTwin,” highlighting ten core functionalities. This approach reduces barriers and accelerates Digital Twin adoption in healthcare, agriculture, and energy sectors.
Challenges and Limitations of Digital Twin Technology
Despite its benefits, the technology faces significant challenges:
- High Initial Investment: Development and implementation require significant resources.
- Cybersecurity: Constant connectivity exposes systems to vulnerabilities.
- Lack of Standardization: Universal standards for developing and operating digital twins are absent.
Addressing these challenges will be essential to fully harness the potential of this technology.
The Future of Digital Twins: Emerging Trends
Digital Twins are evolving with trends like:
- Integration with 5G: Enhances data speed and accuracy.
- Hybrid Digital Twins: Combine multiple systems into a single model. Wang et al., (2024) propose the concept of the Human Digital Twin (HDT) to realize human-centric smart manufacturing in Industry 5.0.
- Sustainability: Digital Twins aid in planning greener, more efficient processes.
In the future, Digital Twins will play a critical role in building smart cities, personalizing products, and simulating complex scenarios, becoming a cornerstone of digital transformation.
Conclusions
Digital twins are tools that aid decision-making by providing digital replicas of processes or systems for simulations.
While developing a digital twin requires a multidisciplinary team of professionals and technicians, its growing use in fields like industry, healthcare, agriculture, and urban planning underscores its necessity. Additionally, research by Robles et al., (2023) has developed the first open-source “open twins” tool to facilitate Digital Twin adoption across industries.
If you enjoyed this article, please share it with your colleagues to support our growth.
References
Björnsson, B., Borrebaeck, C., Elander, N. et al. Digital twins to personalize medicine. Genome Med 12, 4 (2020). https://doi.org/10.1186/s13073-019-0701-3
Boschert S., Rosen R. (2016) Digital Twin—The Simulation Aspect. In: Hehenberger P., Bradley D. (eds) Mechatronic Futures. Springer, Cham. https://doi.org/10.1007/978-3-319-32156-1_5
Chow A. 2021. How Digital Twins Are Transforming Manufacturing, Medicine and More
Crawford M. 2021. 7 Digital Twin Applications for Manufacturing. The American Society of Mechanical Engineers.
Esteban J. 2021. Seys Talks: Gemelos Digitales y BIM, el futuro para la gestión y mantenimiento de infraestructuras
Glaessgen E. and D. Stargel. 2012. The digital twin paradigm for future nasa and us air force vehicles. In 53rd AIAA/ASME/ASCE/AHS/ASC Structures, Structural Dynamics and Materials Conference 20th AIAA/ASME/AHS Adaptive Structures Conference 14th AIAA, page 1818.
Grieves M. 2014. Digital twin: Manufacturing excellence through virtual factory replication. White paper.
Hartmann D., Van der Auweraer H. (2021) Digital Twins. In: Cruz M., Parés C., Quintela P. (eds) Progress in Industrial Mathematics: Success Stories. SEMA SIMAI Springer Series, vol 5. Springer, Cham. https://doi.org/10.1007/978-3-030-61844-5_1
IBM. ? What is a digital twin?
Jiang Yuchen, Yin Shen, Li Kuan, Luo Hao and Kaynak Okyay. 2021. Industrial applications of digital twins. Phil. Trans. R. Soc. A.3792020036020200360 http://doi.org/10.1098/rsta.2020.0360
Lima AC, Royer E, Bolzonella M et al. Digital twins for land-based aquaculture: A case study for rainbow trout (Oncorhynchus mykiss) [version 1; peer review: awaiting peer review]. Open Research Europe 2022, 2:16.
Preut, Anna, Jan-Philip Kopka, and Uwe Clausen. 2021. “Digital Twins for the Circular Economy” Sustainability 13, no. 18: 10467. https://doi.org/10.3390/su131810467
Pylianidis C., Sjoukje Osinga, Ioannis N. Athanasiadis. 2021. Introducing digital twins to agriculture, Computers and Electronics in Agriculture, Volume 184, 105942, ISSN 0168-1699, https://doi.org/10.1016/j.compag.2020.105942.
Robles Julia, Cristian Martín, Manuel Díaz. 2023. OpenTwins: An open-source framework for the development of next-gen compositional digital twins, Computers in Industry, Volume 152, 2023, 104007, ISSN 0166-3615, https://doi.org/10.1016/j.compind.2023.104007.
Soori, M., Arezoo, B., & Dastres, R. (2023). Digital twin for smart manufacturing, A review. Sustainable Manufacturing and Service Economics, 2, 100017. https://doi.org/10.1016/j.smse.2023.100017
Tao F. and Q. Qi. 2019. Make more digital twins. Nature.
Tao, F., Xiao, B., Qi, Q., Cheng, J., & Ji, P. (2022). Digital twin modeling. Journal of Manufacturing Systems, 64, 372-389. https://doi.org/10.1016/j.jmsy.2022.06.015
TAO, F., SUN, X., CHENG, J., ZHU, Y., LIU, W., WANG, Y., XU, H., HU, T., LIU, X., LIU, T., SUN, Z., XU, J., BAO, J., XIANG, F., & JIN, X. (2024). MakeTwin: A reference architecture for digital twin software platform. Chinese Journal of Aeronautics, 37(1), 1-18. https://doi.org/10.1016/j.cja.2023.05.002
Vallée, A. (2023). Digital twin for healthcare systems. Frontiers in Digital Health, 5, 1253050. https://doi.org/10.3389/fdgth.2023.1253050
Van Der Auweraer H, Stijn Donders, Dirk Hartmann, and Wim Desmet. 2018. Simulation and digital twin for mechatronic product design. In Proceedings of ISMA 2018-International Conference on Noise and Vibration Engineering and USD 2018-International Conference on Uncertainty in Structural Dynamics, pages 3547–3565
Verdouw C., Bedir Tekinerdogan, Adrie Beulens, Sjaak Wolfert. 2021. Digital twins in smart farming, Agricultural Systems, Volume 189, 2021, 103046, ISSN 0308-521X, https://doi.org/10.1016/j.agsy.2020.103046.
Voigt Isabel, Inojosa Hernan, Dillenseger Anja, Haase Rocco, Akgün Katja, Ziemssen Tjalf. 2021. Digital Twins for Multiple Sclerosis. Front. Immunol., 03 May 2021 | https://doi.org/10.3389/fimmu.2021.669811
Wang, B., Zhou, H., Li, X., Yang, G., Zheng, P., Song, C., Yuan, Y., Wuest, T., Yang, H., & Wang, L. (2024). Human Digital Twin in the context of Industry 5.0. Robotics and Computer-Integrated Manufacturing, 85, 102626. https://doi.org/10.1016/j.rcim.2023.102626
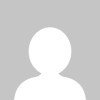
Editor and founder of “Innovar o Morir” (‘Innovate or Die’). Milthon holds a Master’s degree in Science and Innovation Management from the Polytechnic University of Valencia, with postgraduate diplomas in Business Innovation (UPV) and Market-Oriented Innovation Management (UPCH-Universitat Leipzig). He has practical experience in innovation management, having led the Fisheries Innovation Unit of the National Program for Innovation in Fisheries and Aquaculture (PNIPA) and worked as a consultant on open innovation diagnostics and technology watch. He firmly believes in the power of innovation and creativity as drivers of change and development.